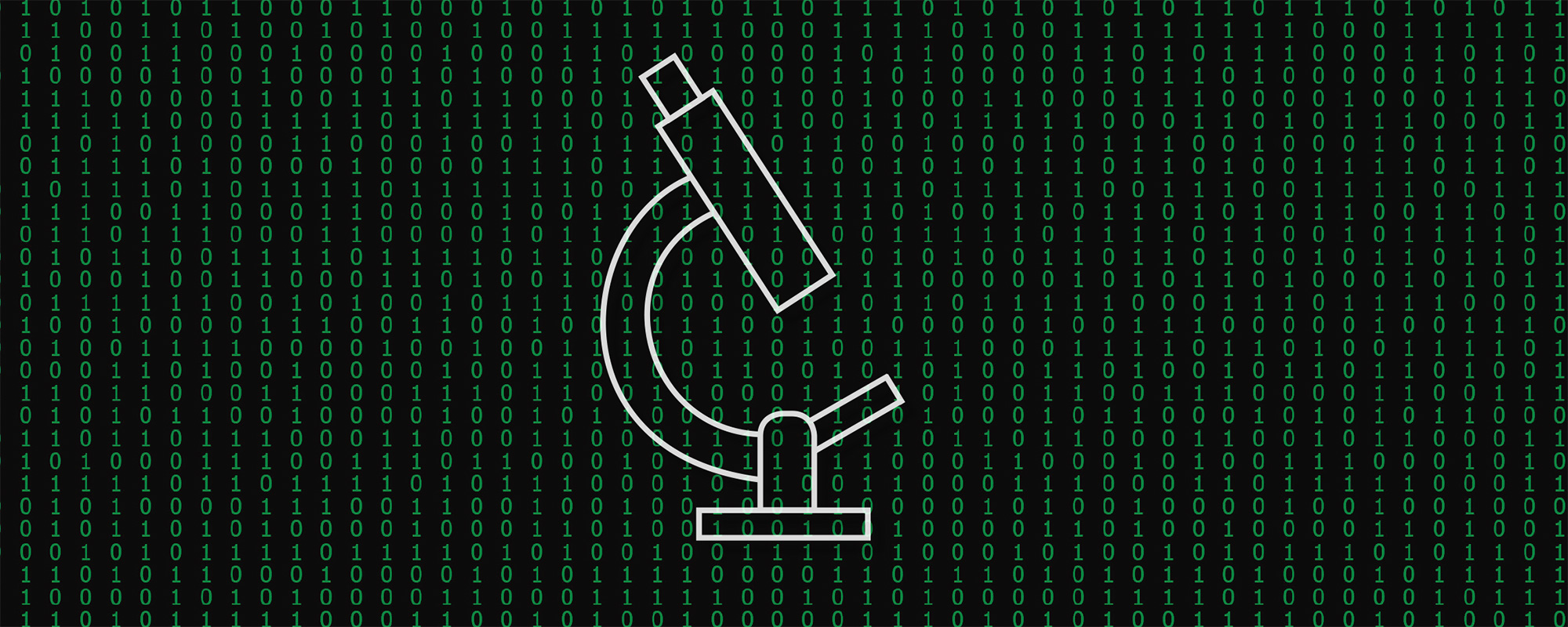
The deluge is upon us.
We are living in the age of big data, and with every link we click, every message we send, and every movement we make, we generate torrents of information.
In the past two years, the world has produced more than 90 percent of all the digital data that has ever been created. New technologies churn out an estimated 2.5 quintillion bytes per day. Data pours in from social media and cell phones, weather satellites and space telescopes, digital cameras and video feeds, medical records and library collections. Technologies monitor the number of steps we walk each day, the structural integrity of dams and bridges, and the barely perceptible tremors that indicate a person is developing Parkinson’s disease. These are the building blocks of our knowledge economy.
This tsunami of information is also providing opportunities to study the world in entirely new ways. Nowhere is this more evident than in medicine. Today, breakthroughs are being made not just in labs but on laptops, as biomedical researchers trained in mathematics, computer science, and statistics use powerful new analytic tools to glean insights from enormous data sets and help doctors prevent, treat, and cure disease.
“The medical field is going through a major period of transformation, and many of the changes are driven by information technology,” says George Hripcsak ’85PS,’00PH, a physician who chairs the Department of Biomedical Informatics at Columbia University Irving Medical Center (CUIMC). “Diagnostic techniques like genomic screening and high-resolution imaging are generating more raw data than we’ve ever handled before. At the same time, researchers are increasingly looking outside the confines of their own laboratories and clinics for data, because they recognize that by analyzing the huge streams of digital information now available online they can make discoveries that were never possible before.”
To date, the most dramatic achievements of data science in medicine have been in the realm of genomics. Physicians at many leading health-care organizations and medical schools, including Columbia’s, now routinely analyze the DNA of their patients, parsing the millions of chemical units that make each one of us unique, in order to more precisely diagnose illness. This has enabled physicians to craft personalized treatments for many forms of cancer, as well as for certain cardiovascular, neurological, pulmonary, and ophthalmological disorders.
But the use of data science in medicine extends far beyond genomics. Today, researchers at CUIMC are using the power of data to identify previously unrecognized drug side effects; they are predicting outbreaks of infectious diseases by monitoring Google search queries and social-media activity; and they are developing novel cancer treatments by using predictive analytics to model the internal dynamics of diseased cells. These ambitious projects, many of which involve large interdisciplinary teams of computer scientists, engineers, statisticians, and physicians, represent the future of academic research.
“Our ability to collect, analyze, and interpret more and larger data sets is infusing new ideas and energy into virtually every academic field today — from data-rich disciplines like astronomy, biology, and climate science to increasingly data-driven professions like law, business, and journalism,” says Jeannette M. Wing, director of Columbia’s Data Science Institute, which supports collaborations between data scientists and researchers in other fields across the University. “Since data is everywhere, data science is applicable everywhere. What’s happening at the medical campus right now represents a kind of collaboration we’re bringing to every corner of Columbia.”
New insights on drug safety
For CUIMC researcher Nicholas Tatonetti, any sizable collection of digital medical records represents a treasure trove of potential discoveries.
Consider, for example, what the young computer scientist has been able to accomplish in recent years by mining an FDA database of prescription-drug side effects. The archive, which contains millions of reports of adverse drug reactions that physicians have observed in their patients, is continuously monitored by government scientists whose job it is to spot problems and pull drugs off the market if necessary. And yet by drilling down into the database with his own analytic tools, Tatonetti has found evidence that dozens of commonly prescribed drugs may interact in dangerous ways that have previously gone unnoticed. Among his most alarming findings: the antibiotic ceftriaxone, when taken with the heartburn medication lansoprazole, can trigger a type of heart arrhythmia called QT prolongation, which is known to cause otherwise healthy people to suddenly drop dead.
“What’s surprising is that neither of those medications had ever been linked to heart problems on its own,” says Tatonetti, an assistant professor of biomedical informatics, systems biology, and medicine. “That’s part of the reason nobody had spotted the risk.”
Tatonetti made the discovery by employing a novel deductive technique: he searched the FDA database for instances of people developing heart problems after taking drugs that aren’t known to cause cardiovascular issues but that share numerous other side effects with medications that are. Then, to assess the strength of the correlations he found, he designed a set of algorithms inspired by an analytic approach called signal-detection theory, which was developed by the US Air Force in the 1940s to help radar operators determine whether objects picked up by their antennas were actually airplanes. These tools enabled Tatonetti to separate the signal from the noise in the FDA archive, accomplishing something that was, to a data scientist, akin to detecting a pea beneath a pile of mattresses.
But Tatonetti didn’t stop there. He then dove into CUIMC’s own patient archive, which contains clinical data on five million patients dating back to 1989. This confirmed that people who had been prescribed ceftriaxone and lansoprazole at the same time often developed irregular heartbeats. Finally, Tatonetti teamed up with Robert Kass, a CUIMC pharmacologist, to undertake a series of experiments to see exactly how ceftriaxone and lansoprazole affect the heart. The results were dramatic: in combination, the drugs were shown to block an electric pathway inside heart cells that controls their pulsing.
“The scope of data collection that went into these studies, and the level of analytic sophistication that was required along the way, is like nothing else I’ve ever seen in the area of drug safety,” says Raymond Woosley, a national expert on QT prolongation who assisted in Tatonetti’s investigation.
Since coming to Columbia straight out of Stanford’s graduate school in 2012, Tatonetti has surprised colleagues time and time again with his ability to glean answers to big, bold questions by trawling collections of digital health records. Last year, he published a comprehensive analysis of how a person’s birth date influences his or her lifetime risk for developing many common health problems. That study, which is based on Tatonetti’s analysis of the medical records of ten million people in the United States, South Korea, and Taiwan, will likely be picked over for years by public-health researchers eager to understand how seasonal environmental conditions — like levels of sunlight, mold, or air pollution — affect pregnant women and their unborn children. And later this year, Tatonetti and several colleagues will publish a groundbreaking analysis of CUIMC medical records that reveals the relative heritability of 467 medical conditions — from anxiety to celiac disease to cystic fibrosis — for which no reliable estimates of heritability have ever been available. The key to the mystery had been staring at researchers for years, right on the hospital intake forms that every patient fills out: the familial relationships of the patients’ emergency contacts.
“We mapped out the relationships of millions of patients and then looked to see the degree to which medical conditions run in families,” says Tatonetti, noting that the results could help researchers identify genes that contribute to disease.
A thirty-five-year-old with a neatly trimmed beard and tattoos on his forearms, Tatonetti is part of a new wave of tech-savvy medical researchers who have largely bypassed traditional investigative approaches, such as observing patients firsthand in clinical studies, in favor of sifting through piles of existing medical data in search of scientific gold. The potential for this kind of research, its proponents say, has grown dramatically in recent years as the health-care industry has fully embraced digital record-keeping: whereas ten years ago the majority of US health-care institutions still relied on paper files to track their patients’ medical care, today only a small percentage of them do.
“The shift toward electronic record-keeping has just totally blown open the possibilities for what you can do as a medical researcher,” says Tatonetti. “A few years ago, if I’d told epidemiologists that I was planning to investigate how a person’s birth month relates to her health, they would have laughed me out of the room.”
Tatonetti came to Columbia, he says, because CUIMC was one of the first medical centers to adopt electronic record-keeping and therefore possesses one of the richest patient databases in the world. Today it contains tens of millions of hospital intake forms, lab results, X-ray reports, prescription orders, immunization records, echocardiograms, vital signs, doctors’ and nurses’ notes, and discharge summaries. Faculty in Columbia’s Department of Biomedical Informatics have pioneered innovative ways of using such data — both to improve patient care and to advance scientific knowledge. On the clinical side, they have developed artificial-intelligence systems that can analyze a patient’s entire medical history within seconds and then alert a CUIMC physician if, for instance, the patient is due for an immunization, is allergic to a medication that he or she is about to be prescribed, or is showing early signs of difficult-to-diagnose conditions like chronic kidney disease. To support new kinds of research, they have created special database-management tools that enable CUIMC officials to share patient data with researchers at Columbia and beyond in ways that protect the patients’ privacy.
“A big priority within the research community right now is figuring out how scientists from different medical centers can pool our data, so that we can all conduct more powerful studies,” says George Hripcsak, the chair of the biomedical-informatics department. He says that CUIMC is at the forefront of efforts to meet this challenge. “We’ve organized a number of national and international consortiums that expand scientists’ access to medical data while at the same time protecting patient privacy.”
The most ambitious of these initiatives, the Observational Health Data Sciences and Informatics program (OHDSI), has created a data-sharing network that enables researchers at academic institutions in twenty-five countries to study the medical records of some four hundred million people, drawn from eighty health-care organizations around the world. Researchers participating in the network, of which CUIMC is the coordinating center, are now mining the records for insights into any number of topics: racial disparities in health-care access, country-by-country differences in how physicians treat common diseases, and problems that arise when children are prescribed adult medications, to name a few. Hripcsak himself is using the archive to assemble what will be a first-of-its-kind catalog revealing the rates at which people who take any of thousands of prescription drugs experience side effects. He says that physicians currently have no way of knowing how frequently many drug side effects occur, because the clinical trials conducted by pharmaceutical companies prior to releasing new drugs — which remain a primary source of scientific information about drug safety today — are too small to accurately assess their prevalence. But Hripcsak believes that by documenting all the health problems that millions of people have experienced shortly after starting on prescription drugs, and then using a number of analytic tricks to weed out incidental correlations in the data, he will be able to provide solid estimates for the prevalence of many drug side effects for the first time.
![]()
Shaman’s team studied information about every case of influenza reported to the CDC since 2003, along with climate data from the same period.
“Does a particular medication carry a 20 percent chance of causing a seizure or a 0.2 percent chance? That difference might determine whether or not you prescribe it to somebody,” he says. “But today, physicians are often in the dark when trying to make these kinds of judgment calls. They’ll read the list of potential side effects on a drug’s label but have little idea what real risk they pose.”
In addition to containing enormous amounts of information of value to physicians and patients, the new catalog could also be a boon for researchers.
“One of the things I’ll be using the catalog for is to spot more dangerous drug combinations,” says Tatonetti. “Knowing the rates at which certain side effects occur will provide us clues as to which pairs of drugs — among the thousands of pairs that may at first glance appear to be troublesome — are the most important to investigate.”
None of this is to say that data mining is going to replace traditional forms of medical research. Both Hripcsak and Tatonetti acknowledge, for example, that the only way to evaluate the safety of new drugs is to see how they work on small numbers of people in closely monitored clinical trials. But they predict that as the insights of big-data analytics are gradually integrated into routine medical practice, with data scientists tapping into the rivers of digital information flowing out of doctors’ offices and sharing their insights with practitioners in real time, a fundamentally different kind of healthcare system will emerge.
“This will create what data scientists like to call a ‘learning health system,’ where medical treatments and procedures can be continuously monitored and tweaked, in accordance with how they’re performing,” says Hripcsak. “Eventually, we’ll also have massive quantities of data coming in from mobile monitoring devices, like smart watches that record your vital signs. By analyzing that data, we could enable a physician to provide you individually tailored medical advice without you even stepping into his or her office.”
Using data to predict flu epidemics
Every year, in the fall or winter, a wave of influenza hits the United States. And every year, health officials struggle to respond, because they don’t know when the flu will strike or what parts of the country will be hardest hit. In a typical flu season, tens of thousands of Americans are killed by the virus, but if the timing and severity of outbreaks could be anticipated, then health officials could respond more effectively and save lives.
Jeffrey Shaman ’03GSAS, an associate professor of environmental health sciences at Columbia’s Mailman School of Public Health, has found a way to predict flu outbreaks using big-data analysis. Originally trained as a climate scientist, Shaman has for the past several years been developing computer systems that can anticipate the timing and magnitude of flu epidemics by analyzing many different types of data, some of which pertain to actual incidences of influenza and others to conditions in which the virus generally likes to spread. A typical forecast produced by his team might declare, for example, that there is a 60 percent chance of the city’s flu season peaking in intensity in five weeks.
“That can give health-care workers more time to prepare,” says Shaman, whose team currently publishes weekly flu forecasts for eighty-one US cities and all fifty states on its Columbia website. “They can stock up on medications like Tamiflu, assign more staff to emergency rooms, and launch public-awareness campaigns to maximize their impact.”
Predicting flu outbreaks has long been a dream of public-health researchers, but until recently scientists knew too little about how influenza spreads. Even the most obvious feature of influenza’s global migration cycle — that it emerges in temperate regions in both the Northern and Southern Hemispheres during cold months — had been difficult to explain.
Shaman achieved a major breakthrough in this area when, in 2008, he discovered that the flu virus is adept at spreading in conditions of low humidity, such as those that prevail in North America during the winter. “No one’s sure why this is, but there are a number of theories that attempt to explain why the flu virus, when expelled from a host as tiny airborne droplets, would be sensitive to ambient humidity,” he says. “Some scientists have speculated that when it’s less humid, chemical changes occur in the droplets that may protect flu viral particles trapped inside and make them more likely to infect people who inhale them.”
Shaman, who studied hydrology and atmospheric sciences for many years before turning his attention to influenza, made this discovery by reanalyzing data that a group of Mount Sinai Hospital virologists had collected in a series of lab experiments that assessed the impact of humidity and temperature on the flu’s transmissibility. The virologists had concluded that these factors had only a modest impact on flu transmission; Shaman, who as an environmental scientist was accustomed to dealing with such data sets, showed that humidity was, in fact, a very important factor.
“Whereas the original authors had looked at the effects of relative humidity, or the amount of water vapor in the air as a percentage of what it can hold at a given temperature, my team looked at the effects of absolute humidity, which is a more straightforward, mass-based measure, and we found that its effects were pronounced,” he says.
Armed with this insight, Shaman and his colleagues began work on a flu forecasting system that was one of the first of its type. In order to train their computer to predict future epidemics, they first downloaded and studied information about every case of influenza reported to the US Centers for Disease Control and Prevention (CDC) since 2003, along with detailed climate data covering the same period. The researchers then developed a computer model capable of making probabilistic predictions based on a steady stream of flu data it would receive from a number of disease-monitoring organizations, including the CDC, and climate data. They also taught the system to incorporate data that Google had just begun releasing daily on the locations and numbers of people searching for flu-related keywords.
“The Google data stream was vital because it gave us nearly instantaneous knowledge about what was happening on the ground,” says Sasi Kandula, a Columbia computer scientist who has contributed to the project. “Traditional epidemiological data, which consists of doctors’ reports of flu cases, is typically a week or two old by the time an organization like the CDC releases it.”
In 2012, after nearly four years spent developing their system, Shaman and his colleagues began releasing real-time flu predictions. The next year, CDC officials evaluated the Columbia team’s predictions along with those produced by five other research groups, and they declared the Columbia team’s the most reliable.
Since then, Shaman and his colleagues have been refining their models. By studying the pace at which influenza spreads through populations of varying densities and cities with different types of infrastructure, for example, they’ve improved the geographic resolution of their predictions to the point where, last winter, they developed a new forecasting system able to specify where in large cities the flu would hit first, down to the level of individual neighborhoods.
At the same time, the researchers have taken their work to the international stage, collaborating with scientists in Hong Kong and several other cities in Southeast Asia to build flu-prediction systems designed specifically for that region. “Forecasting flu outbreaks in this part of the world is important, since new and dangerous strains of the virus often emerge there,” says Wan Yang, a Columbia epidemiologist, environmental engineer, and computer scientist who is working on the project.
In the US, meanwhile, Shaman’s team is attempting to plug some major gaps in our knowledge of how influenza spreads from person to person. One possibility that has long kept epidemiologists awake at night, Shaman says, is that some people carrying the flu virus may not develop symptoms and therefore go about their days blithely infecting others. The winter before last, Shaman and his colleagues, as part of a federally funded study, began collecting nasal swabs from large numbers of people in schools, daycare centers, and other public places in New York City.
“We’re on the lookout for people who aren’t visibly sick, yet are shedding the virus,” says Shaman.
He says that if significant numbers of asymptomatic people are found to be contagious, this might prompt city health officials to proactively screen people for influenza. No matter what he and his colleagues discover through swab sampling, Shaman says, the study will move them one step closer to their ultimate goal, which is gaining a comprehensive understanding of how influenza moves through populations.
“Right now, flu forecasting is probably at the point where weather forecasting was fifty years ago,” says Shaman, who notes that his forecasts are used only informally by health officials. “But as we develop better, more sophisticated influenza surveillance, and as we’re better able to assimilate all the available data, that situation is going to change very quickly.”
Unlocking the power of citizen scientists
A woman who complains to her doctor about extreme menstrual pain is likely to be told, It’s a normal part of being a woman, so tough it out.
And yet, too often, the pain is not normal: it’s the result of a disease called endometriosis, which occurs when uterine cells migrate outside the uterus, forming lesions that glom onto other organs. Experts say that this condition, which can damage the reproductive system if left untreated, often goes undiagnosed, because its primary symptom is an intense pelvic pain that occurs around the same time as a woman’s period. According to many women’s health advocates, the tendency of physicians to dismiss this pain as ordinary menstrual cramps has perpetuated a cycle of misinformation about endometriosis, with the medical establishment viewing it as an uncommon disorder and therefore investing little money in its research.
Three years ago, Noémie Elhadad ’06SEAS, a Columbia medical researcher who suffers from endometriosis, decided to take matters into her own hands. A computer scientist who specializes in wresting insights from messy data sets, like collections of doctors’ notes or patients’ comments in online forums, she figured that if no major funding was available for a study on endometriosis, she’d come up with a technological hack to conduct one on the cheap. And one night while participating in a patient support group with fellow “endo” patients, as women with the disease call themselves, she had an idea for how to do it.
“I noticed that a lot of women were using smartphone apps that track your menstrual cycle, based on information you enter about any cramping, bloating, or bleeding you experience each day,” says Elhadad. “And I thought, why don’t we design a similar tool for women with endometriosis? Then they can document the nuances of their condition as citizen scientists.”
Elhadad realized her vision last year, launching a crowdsourcing project called Citizen Endo. At the heart of the effort is a smartphone app, Phendo, that Elhadad developed with a $50,000 grant from the Endometriosis Foundation of America. The project has already amassed the largest collection of clinical data about endometriosis in existence. Nearly three thousand endometriosis patients in sixty-five countries have used the app on a daily basis, some for several months at a stretch, to document their pain, energy levels, moods, diet, physical activities, medications, and pain-management strategies. The data is then transmitted to a computer in Elhadad’s office at CUIMC, where she and the members of her research team analyze it for insights into how the disease manifests in different women.
“A lot of the women choose to participate simply because they’re passionate about helping to push the science forward,” says Elhadad.
The goal of the project, Elhadad says, is to describe the full range of endometriosis’s symptoms, and thereby help physicians diagnose and treat more cases. (The disease is typically treated with laparoscopic surgery to remove the lesions and hormonal therapy to prevent their regrowth.)
“Today, a doctor who’s trying to diagnose endometriosis doesn’t have a lot of information to go on,” says Elhadad, noting that previous studies on the disease have been too small to provide a proper accounting of its symptoms. “And plenty of the information that is available, we’re finding out now, is just plain wrong.”
Consider, for example, what the current medical literature says about the pain endured by endometriosis patients. A seminal paper on the topic, published by Harvard scientists in 2002, suggests that the pain is restricted to the pelvic region. But that’s not true, according to Elhadad. She says that her data indicates that more than half of all women with the disease have pain that radiates down their back, arms, or legs — sometimes in combination with pelvic pain and sometimes without it. And while the Harvard paper states that endometriosis pain always strikes women in sync with their periods, Elhadad’s data reveals that many endometriosis patients suffer chronic pain that can persist for months or even years.
“It’s actually been common knowledge for quite some time now among women with the disease, and some savvy gynecologists, that the pain can persist outside of a woman’s period,” she says. “But ours is the first study to document it.”
![]()
In less than two years, Citizen Endo has amassed the largest collection of clinical data about endometriosis in existence.
Other findings were completely unexpected. After asking to see their subjects’ medical histories, for example, Elhadad and her colleagues, who include biomedical informatics PhD candidate Mollie McKillop ’14PH, discovered that many of the women had a history of urinary problems, such as incontinence or painful urination, not previously linked to endometriosis. The researchers are now scouring the data they received via the smartphone app to determine, for example, if a history of urinary issues may be linked to the severity of the disease, responsiveness to certain pain-management strategies, or a woman’s chances of suffering what is perhaps the most feared outcome of the disease: infertility.
“We know already that about half of all women with endometriosis lose their ability to have children, often at a very young age, but we can’t predict who this will happen to,” Elhadad says. “I’ve heard twenty-two-year-old women say things like, ‘Well, I don’t really want to have a child right now, but maybe I should start trying before it’s too late.’ That’s a horrible situation to be in. But if we can identify those patients who are likely to become infertile, we could share that information and help them make better choices.”
The Columbia researchers say they’re still at the beginning of their evidence-gathering journey. Later this year, they will enroll an additional seven thousand women in Citizen Endo. They are also planning to expand the scope of their project to eventually incorporate analyses of their subjects’ hormonal profiles, which they would acquire by having women submit blood or saliva samples. There’s no end to the discoveries this effort could yield, the researchers say, since scientists currently know so little about endometriosis. Among the questions they hope to investigate are what causes the disease; whether it might be treated without surgery; and how prevalent it is (some gynecologists have estimated that 6 to 10 percent of all women may have endometriosis, although they say this assessment is very speculative).
“Just about anything we learn is going to be valuable, because we’re starting from a place of such ignorance,” says Elhadad, noting that women with the disease currently go an average of seven years before being diagnosed.
Elhadad suspects that women are enthusiastic about participating in Citizen Endo because they’re grateful that medical professionals are now listening to them. And she says that she hopes to make their efforts more rewarding by eventually adding new features to her smartphone app to give women individualized tips on how best to manage their condition.
“One of the wonderful things about mobile technology today is that medical researchers and study subjects can communicate back and forth in ways that benefit everybody,” she says. “I mean, sure, I now have access to huge amounts of information about these women’s daily lives. But I need to give them back something in return. And what I’m going to give them should be the most personalized, intimate, and timely health advice they’ve ever received about their condition.”
Computing vs. cancer
It has been nearly forty years since scientists discovered that cancer is caused by flaws in our DNA, and that insight still guides most oncology research today, inspiring scientists to hunt for cancer-causing genes and to search for drugs that help people with particular mutations.
Andrea Califano, the founding director and chair of CUIMC’s Department of Systems Biology, has taken a different approach to studying the disease.
Rather than relying on genetic mutations as signposts in his quest to understand cancer, Califano has plunged headlong into the messy interior dynamics of cancer cells, attempting to determine how the tens of thousands of proteins operating inside cells can conspire to make them divide uncontrollably. It is an approach that has required him to build one of the most complex, data-intensive mathematical models of cellular activity in existence — yet it is revealing that cancer may be a simpler and more treatable disease than we first thought.
“What my team is doing is akin to dismantling a car that’s broken down and then rebuilding it, one piece at a time, in hopes of diagnosing the problem,” says Califano, a former theoretical physicist who worked for several years as a computational biologist at IBM’s Thomas J. Watson Research Center before coming to Columbia in 2003. “We think this may be the only way we’ll ever truly understand how a cancer cell works.”
Califano set out on this path about ten years ago, when cancer researchers were beginning to realize, after years spent hoping that the Human Genome Project would produce a clear road map for fighting cancer, that the disease involves far more genes than anyone had previously imagined. Although a handful of genetic mutations wield a strong influence in causing some types of cancer — thereby giving researchers clues to developing new, personalized treatments — most forms of the disease turn out to involve dozens, or even hundreds, of mutations, each contributing a small portion of a person’s overall risk. To make matters more confusing, the genes at the roots of cancer vary considerably from one person to the next, even among people whose tumors start in the same organ and otherwise look identical.
“So this raised the question: is cancer not one disease but actually thousands of different diseases that we’d have to cure individually?” says Califano. “My hunch, and my hope, was that this wasn’t the case. I still believed there had to be some common cellular mechanisms shared by many cancers that we just hadn’t noticed yet. And I thought to find them, we’d have to look beyond genes — straight into the guts of the cell.”
To many biologists, this seemed like an exercise in futility. No practical methods of studying the inner dynamics of entire cells existed at the time; biologists who studied interactions among proteins therefore restricted their analyses to small groups of molecules extracted from cells. Moreover, many biologists thought that diseased cells would be especially difficult to study, since their interior mechanics were going haywire.
“I never bought that idea,” says Califano. “Maybe it’s my background as a physicist, but I tend to assume that nature is operating as efficiently as possible unless evidence tells us otherwise. I saw no reason to suspect that cells with virtually identical capabilities of spreading rapidly throughout your body aren’t operating in an extremely orderly and consistent manner.”
It turns out that he may be right. In a series of stunning papers published over the past few years, Califano and several members of his lab have identified dozens of proteins that they say act as “master regulators” in cancer cells, seamlessly orchestrating the activities of hundreds of other proteins, which, in turn, force the cells to divide and persist in a malignant state. Califano’s team has accomplished this using a sophisticated investigative strategy, which involves measuring the activity levels of all the proteins in large numbers of healthy and cancerous cells; determining which proteins are capable of binding to one another; mapping out all their potential relationships in gigantic sunburst-shaped charts; and then training a computer algorithm to identify which proteins are most influential in making a cell cancerous. It took one of the largest supercomputers in the world, built under Califano’s oversight at CUIMC in 2008, to perform the calculations.
The therapeutic implications of these discoveries could be profound. Califano says that the cancer-driving proteins that he and his colleagues have identified are active in certain subsets of people with many different types of cancer — an assessment based on their analysis of cells drawn from more than twenty thousand patients from across the United States. The researchers have also conducted experiments on mice to determine which of approximately 120 FDA-approved drugs and 340 experimental compounds are most effective against cancer cells that contain heightened levels of these proteins; based on the results, they’ve developed a computer-based diagnostic system that recommends treatment strategies for cancer patients who test positive for the proteins.
“Often, the recommendations are for drugs that no physicians would have ever even thought to use for a certain kind of cancer,” says Califano. “The system can reveal that someone with brain cancer needs the same medication as someone with lung cancer or someone with leukemia. This is because some of the master regulators we’ve identified crop up in all sorts of cancers that nobody knew had underlying similarities.”
To date, Califano’s diagnostic technology has been used in only a handful of cases, when terminally ill cancer patients in the final stages of the disease sought experimental treatments. But the results have been so promising — with some patients having had their lives extended by six months or longer — that the FDA recently approved a clinical study in which dozens of men and women with pancreatic cancer will have their protein levels assessed by Califano’s team during their initial phase of treatment. Califano and his colleagues will then identify a handful of drugs that might help each patient and then work closely with scientists in the laboratory of CUIMC pancreatic-cancer specialist Kenneth Olive to test their effectiveness in mice that have been injected with the patient’s own cancer cells.
“While we’re performing these individually tailored experiments on mice, the patients will receive traditional care,” Olive says. “And then, based on the response of a person’s mouse avatar, we will select which one, among a dozen additional drugs, should be given to the patient.”
Califano hopes the technology, if it proves successful, will be widely used one day in conjunction with DNA tests — thus marrying the best cancer diagnostics of the genetics era and the emerging age of high-powered protein analysis.
“The best cancer care is going to result from bringing together genetics, proteomics, and other novel approaches like immunotherapy,” Califano says. “We must embrace cancer as a highly complex disease and throw everything we have at it.”
Using Data for Good
So what does it take to be a data scientist? Advanced skills in computer science, statistics, or math is a sound start — but it’s only a start. Intellectual versatility is essential, since data scientists often collaborate with experts in fields as varied as business, medicine, law, finance, journalism, and urban planning. And then there is the need to navigate the tricky ethical implications of one’s work. Are data scientists prepared to ensure the responsible use of data through the entire data life cycle, from collection to analysis to interpretation? Or might a project jeopardize people’s privacy, as occurred when the political-consulting firm Cambridge Analytica misused data from tens of millions of Facebook profiles in the lead-up to the 2016 presidential election?
Jeannette M. Wing, the director of Columbia’s Data Science Institute, says that she came to Columbia last year in part to promote discussion among faculty and students about these types of complex issues. A former corporate vice president of Microsoft Research, she notes that the nascent field of data science, which she defines elegantly as “the extraction of value from data,” has yet to establish best practices for handling such challenges. And she thinks that Columbia, which created its Data Science Institute in 2012 — years before similar research centers began to pop up at other universities — is poised to lead the conversation.
“In addition to being five years ahead of the curve in promoting interdisciplinary data-science projects, Columbia has an advantage in that lots of our scholars in the social sciences and humanities want to be a part of this dialogue,” she says. “And if the field of data science is going to evolve in a socially responsible way, you have to include their perspectives.”
Wing has certainly succeeded in raising the visibility of data science at Columbia since arriving here. The Data Science Institute, initially based in the engineering school, has been elevated to a University-wide research center; its 250 affiliated faculty and researchers are engaged in projects that touch nearly every academic discipline. Wing has also launched a postdoctoral fellowship program in data science, an undergraduate research program for promising young talent in the field, a seed-grant program to support new research collaborations, and a fundraising initiative aimed at creating new data-science faculty positions.
In all of her efforts, Wing says, she is guided by a simple mantra: “data for good.”
“I always say that at Columbia, we are harnessing the power of data science across all fields to drive exploration, provide insights, and make predictions to inform better decisions,” she says. “‘Data for good’ means using the power responsibly and ethically to tackle society’s greatest challenges.”